Anomaly detection
Anomaly detection uses a large number of normal examples to train an algorithm which detects what is normal (based on the training examples) and what is not normal. Anomaly detection has features of both supervised and unsupervised learning, and is applicable to Radiology as it’s important to differentiate the normal from the abnormal
Anomaly detection is especially useful when there are many different “types” of anomalies as it’s hard for any algorithm to learn from examples what different types of anomalies should look like and future anomalies may not show similarities to anomalies in any training data.
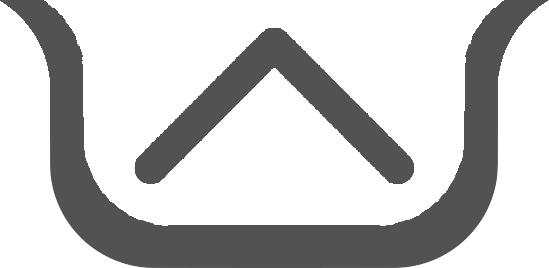