Overfitting
Overfitting is a problem in machine learning that introduces errors based on noise and meaningless data into prediction or classification. Overfitting tends to happen in cases where training data sets are either of insufficient size or training data sets include parameters and/or unrelated features correlated with a feature of interest non-randomly. For example, an algorithm trained to read chest x-rays may correlate the use of a side marker or absence of a side marker with the absence/non-absence of pathology.
Strictly speaking, overfitting applies to fitting a polynomial curve to data points where the polynomial suggests a more complex model than the accurate one. In terms of neural networks, classification results which are misclassified by irrelevant parameters are referred to as examples of overfitting.
There are many techniques to correct for overfitting including regularization.
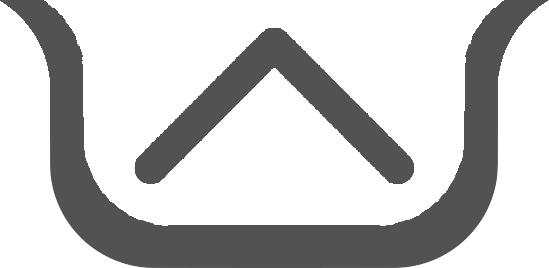