Power
Power is a critical concept when planning or evaluating a radiology study:
- power = (1 - β)
Conventionally, power is set at 0.80-0.85.
Concept
When reviewing a sample data set, the mean of the value in question of the experimental population is likely different from the overall population. In other words, after you do something to your experimental sample, you expect the variable you're watching to change.
For instance, imagine you want to evaluate the size of the pancreatic duct after administering secretin. You think secretin will increase the size of the pancreatic duct. Pathologic data is your gold standard, and using this technique, it's been shown that an average adult patient has a mean size duct (μ) with a standard deviation (σ). Now you give a sample population a bolus of secretin and start counting...
The mean size of the pancreatic duct from the post-secretin experimental population (X) is greater than expected from the path data... but is this a real effect or is this just due to chance?
If we set the p-value to 0.05, then we know we have only a 5% chance of making a type I error (α)... the error of saying that the increase in size of the pancreatic duct is a real effect, when it really isn't.
If our study does not show that the increase is significant to the 0.05 level, then we cannot believe that secretin made a difference, but we run the risk of a type II error (β)... the error of saying that there is no difference when there really is.
The question then becomes, how do we know we have enough people in our experimental group to show a difference if there were one? This is how you power a study effectively. If the sample size is too small (underpowered), then the risk of a type II error increases.
You can imagine two tests for the pancreatic duct: one with 15 post-secretin patients and one with 115 post-secretin patients. Both may fail to meet the p-value, but intuitively we know that the second test did a better job of trying to show a difference if there were one. This is what we're trying to capture with the concept of power.
The key variables in power are
- the size of the difference you expect between the two groups
- the smaller the difference you expect between the experimental and control group, the larger number of subjects you need to tease out that small difference
- the number of patients
- the variability of the data, as captured by the standard deviation
- the more variable (noisy) the data, the more patients you will need to show a difference
- the alpha cut off (usually 0.05)
Post hoc power analysis
The use of post hoc power analysis (i.e. calculating power after the study has concluded) is controversial as it is thought to be unreliable .
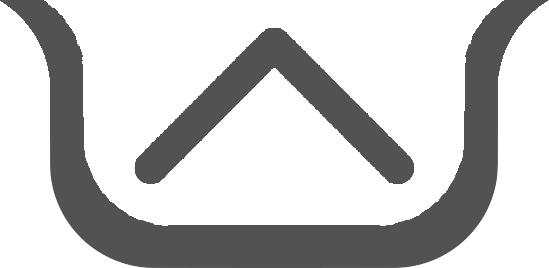