Texture analysis
Texture analysis is a non-invasive, mathematical method assessing the spatial heterogeneity of regions of interest in medical imaging, its primary application is in the assessment of tumors. Although not a new topic of research, the past decade has seen a significant resurgence of texture analysis in the field of radiomics .
Traditionally, the interpretation of tumor bodies in medical imaging whether that be CT, MRI or x-ray will report on the size and parameter metrics . It is now well known that intratumor heterogeneity is a marker of malignancy; texture analysis attempts to provide a comprehensive quantitative analysis of heterogeneity via the assessment of pixels and voxels within a tumor image .
Statistical based modelling
Texture analysis employs a plethora of models to achieve an accurate assessment of tumor heterogeneity, including model-based, transform-based, and statistical-based . The utilization of statistical based modelling is the most common in texture analysis, involving three orders of measure parameters; first-order statistics, second-order statistics and higher-order statistics.
First-order statistics
Explores the frequency distribution in the region of interest via a histogram, it does not consider pixels around the region of interest, the first order statistics measures parameters such as intensity, standard deviation, skewness, and kurtosis .
Second-order statistics
Explores via a run-length matrix, co-occurrence measurements assessing a length of pixels consecutively that have equal grey-level intensities. This will provide information regarding the texture of the region of interest. Fine texture will have shorter run lengths and a more consistent range of intensities and less fine, coarse regions having an opposite read .
Second order statistics via a grey-level co-occurrence matrix will explore similar parameters, however, will present how often pairs of pre-determined pixel values arise within a spatial range in the image.
Higher-order statistics
Explores the overall differences between pixels or voxels within the context of the entire region of interest, often via the utilization of neighborhood grey-tone-difference matrix. Using higher order statistics one can obtain metrics such as variations within the image and the spatial rate of grey-level change. Higher order statistics provides a broader overall report of the region of interest texture metrics .
Clinical applications
Clinical applications studies are substantial, and beyond the realm of this article, however as with all emerging technology/research, clinical validation is a priority .
The utilization of texture analysis presents a non-invasive method to identify and characterize tumors using conventional cross-sectional imaging such as CT and MRI. It enhances the characterization of tumor bodies using complex algorithms and has the potential to overcome the challenges of biopsy .
Texture analysis has also been explored to better characterize hepatic fibrosis, emphysema, and liver cirrhosis, although this research is also still in the experimental phase .
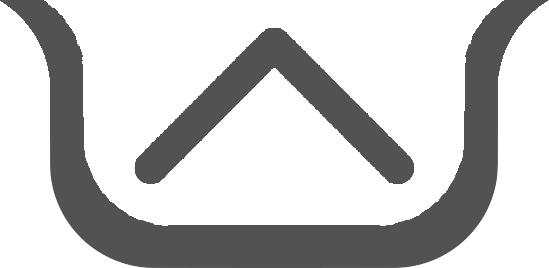