Bagging
Bagging is a term often used in the fields of machine learning, data science and computational statistics that refers to bootstrap aggregation. Bootstrapped aggregation of data can be employed in many different AI (artificial intelligence) algorithms, and is often a necessary step to making random forest algorithms. The term 'bootstrapping' in machine learning does not refer to all kinds of "statistical bootstrapping," an often pejorative term which can imply adding data points to a data set which is too small or a specific group of re-sampling techniques. The term 'bootstrapping' in the context of machine learning refers to a process of repeated re-sampling of data to create subsets of data for specific uses in Machine learning, often to create decision trees.
Bagging is the assembly of re-sampled data subsets to inform a model or algorithm, using the aggregated data (often in the form of summed decision tree outputs) to facilitate decisions. Bagging is therefore considered to be an ensemble method of machine learning.
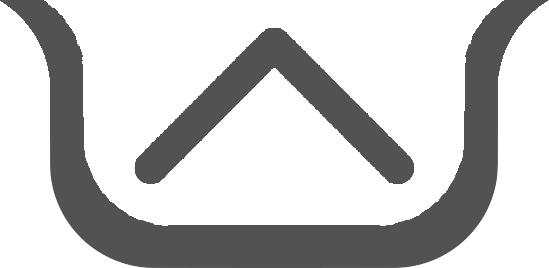