Single linear regression
Single linear regression, also known as simple linear regression, in statistics, is a technique that maps a relationship between one independent and one dependent variable into a first-degree polynomial. Linear regression is the simplest example of curve fitting, a type of mathematical problem in statistics.
Linear regression creates a very limited model because it represents a relationship as a straight line (first-degree polynomial). A first-degree polynomial can describe various different data distributions without giving insight into the true relationships between the variables, which may be better described by a higher degree polynomial. Strictly speaking, many statisticians would consider the linear regression model inapropriate for data that do not meet certain criterion including approximate linearity in the correlation of two continous variables, and a normal distribution of residuals.
Another limitation of the linear regression model is that real-world problems usually involve multiple variables which affect a dependent variable. In the case that multiple variables affect a dependent variable, a multiple regression analysis may be a more precise in describing the relationships of the variables. Of note, an R statistic may be useful to examine how much variability in a dependent variable can be explained by one independent variable.
Related Radiopaedia articles
Research
- clinical trials
- descriptive studies
- statistics
- concepts
- analyzes of variance
- student t-test
- paired t-test
- ANOVA
- one-way ANOVA
- factorial ANOVA
- repeated measures ANOVA
- multivariate ANOVA (MANOVA)
- regression
- single linear regression
- multiple regression analysis
- multiple linear regression analysis
- multiple logistic regression analysis
- non-parametric statistics
- bias
- cognitive bias in image perception
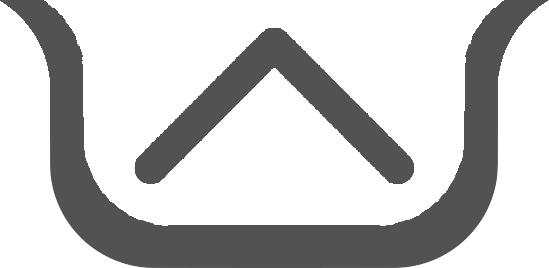