Mean squared error
Mean squared error is a specific type of loss function. Mean square error is calculated by the average, specifically the mean, of errors that have been squared from data as it relates to a function ( often a regression line).
The utility of mean square error comes from the fact that squared numbers are positive, and that errors are squared before they are averaged. Although the absolute value of errors is also always positive, the mean square error has unique characteristics.
By using the square of errors, all values for error are translated into positive numbers. Data with a lot of variances that have values higher and lower than those determined by a function may have a mean error which underestimates the variance or even shows a mean error of zero, as positive and negative values can sum to zero. In order to get a better picture of the variance and bias in data sets, the mean squared error is often used as a measure.
Mean squared error and the root of mean squared error, can for many data sets show variance better than a simple absolute value of errors.
Where n is the number of values in a data set and we presume the error to be the distance from a function representing true values, the mean squared error can be calculated by 1/n (Σ errors ).
Mean squared errors are useful not only in machine learning, but many problems where there is a need to quantify the amount of lack of precision through statistical tools.
Related Radiopaedia articles
Artificial intelligence
- artificial intelligence (AI)
- imaging data sets
- computer-aided diagnosis (CAD)
- natural language processing
- machine learning (overview)
- visualizing and understanding neural networks
- common data preparation/preprocessing steps
- DICOM to bitmap conversion
- dimensionality reduction
- scaling
- centering
- normalization
- principal component analysis
- training, testing and validation datasets
- augmentation
- loss function
- optimization algorithms
- ADAM
- momentum (Nesterov)
- stochastic gradient descent
- mini-batch gradient descent
- regularisation
- linear and quadratic
- batch normalization
- ensembling
- rule-based expert systems
- glossary
- activation function
- anomaly detection
- automation bias
- backpropagation
- batch size
- computer vision
- concept drift
- cost function
- confusion matrix
- convolution
- cross validation
- curse of dimensionality
- dice similarity coefficient
- dimensionality reduction
- epoch
- explainable artificial intelligence/XAI
- feature extraction
- federated learning
- gradient descent
- ground truth
- hyperparameters
- image registration
- imputation
- iteration
- jaccard index
- linear algebra
- noise reduction
- normalization
- R (Programming language)
- Python (Programming language)
- segmentation
- semi-supervised learning
- synthetic and augmented data
- overfitting
- transfer learning
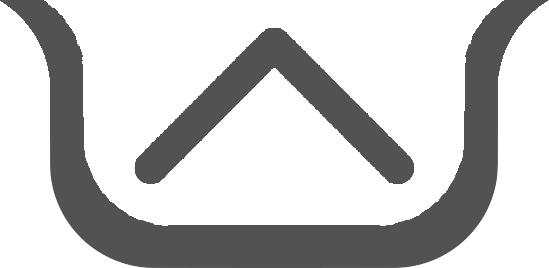