Neural network architectures
Artificial neural networks can be broadly divided into different architectures, feedforward or recurrent neural architectures.
Feedforward neural networks are more readily conceptualised in 'layers'. The first layer of the neural network is merely the inputs of each sample, and each neuron in each successive layer is connected to a set of neurons in the preceding layer.
To compute the function represented by the network, we calculate the activation in each neuron by applying a non-linear activation function (typically a sigmoid function) to the weighted sum of the activations of the connected neurons in the preceding layer. These weights represent the information stored by the neural network and are the parameters that we update during training. The activations of the final layer are the output of the network.
The different choices of how we connect neurons in successive layers to the previous layers strongly influence the abilities of the network and consists of what we normally refer to as the 'architecture' of the network. Common architectures are fully connected neural network and convolutional neural networks.
Related Radiopaedia articles
Artificial intelligence
- artificial intelligence (AI)
- imaging data sets
- computer-aided diagnosis (CAD)
- natural language processing
- machine learning (overview)
- visualizing and understanding neural networks
- common data preparation/preprocessing steps
- DICOM to bitmap conversion
- dimensionality reduction
- scaling
- centering
- normalization
- principal component analysis
- training, testing and validation datasets
- augmentation
- loss function
- optimization algorithms
- ADAM
- momentum (Nesterov)
- stochastic gradient descent
- mini-batch gradient descent
- regularisation
- linear and quadratic
- batch normalization
- ensembling
- rule-based expert systems
- glossary
- activation function
- anomaly detection
- automation bias
- backpropagation
- batch size
- computer vision
- concept drift
- cost function
- confusion matrix
- convolution
- cross validation
- curse of dimensionality
- dice similarity coefficient
- dimensionality reduction
- epoch
- explainable artificial intelligence/XAI
- feature extraction
- federated learning
- gradient descent
- ground truth
- hyperparameters
- image registration
- imputation
- iteration
- jaccard index
- linear algebra
- noise reduction
- normalization
- R (Programming language)
- Python (Programming language)
- segmentation
- semi-supervised learning
- synthetic and augmented data
- overfitting
- transfer learning
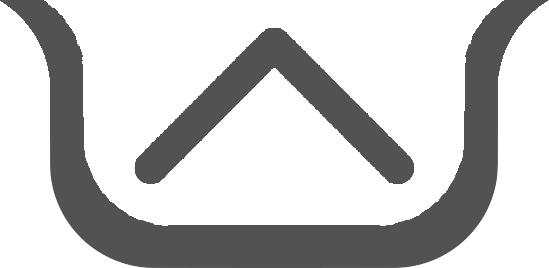